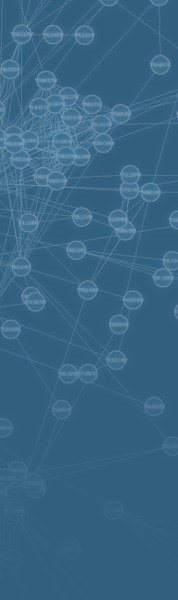
|
|
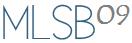 |
|
|
Program
Diego di Bernardo, University of Naples "Federico II", Italy
Networking genes and drugs: Understanding drug mode of action and gene function from large-scale experimental data
A cell can be described as a synergistic ensemble of biological entities (mRNA, proteins, ncRNA, metabolites, etc) interacting with each other, whose collective behaviour causes the observed phenotypes. A great research effort is ongoing in identifying and mapping the network of interactions among biomolecules in mammalian species.
The idea of harnessing this network to understand human diseases at the molecular level, and possibly to find suitable drugs for their treatment, is fascinating but still unfulfilled.
We will show how it is possible to harness experimental data on human cells and tissue to identify the gene regulatory networks among tens of thousands of genes, and how to use this information to analyse the modular structure of the cell and predict the function of each gene. Moreover, we will show how using these data it is also possible to identify a suitable drug, or a combination of drugs, that can restore the physiological behaviour of the affected pathways in human diseases.
Roman Jerala, National Institute of Chemistry and University of Ljubljana, Slovenia
Synthetic Biology: achievements and prospects for the future
Synthetic biology, which combines engineering approach in biological systems is getting a strong momentum due to the recent technological advances, which allow us to manipulate the genetic information at an unprecedented scale.
Currently synthetic biology is exploiting its potentials and advantages but also bottlenecks.
We will review some success stories of synthetic biology in different field of applications, such as medicine, energy and materials. Medical applications of synthetic biology are some of the most promising areas of synthetic biology, particularly for the alternative methods of drug production, biosensors and also different therapeutic applications. Recent developments in our understanding of cellular signaling and host-pathogen interactions provide the opportunity for new types of medical intervention, where we can utilize parts of the existing or reengineer signaling responses connected to various pathological conditions. Knowledge of the ways that microbes use to avoid the human immune response allows us to devise approach to bypass those microbial strategies.
We will look at three different applications of synthetic biology, which involve reengineering of cell signaling pathways, which we have prepared for the international genetically engineered machines competition in years 2006-2008. We have designed and demonstrated proof of the concept of antiviral detection and defense system based on essential viral functions that is independent on mutations and a synthetic vaccine that activates both innate and adaptive immune response.
Nick Juty, European Bioinformatics Institute, UK
Ontologies for systems biology
he ease with which modern computational and theoretical tools can be applied to modeling has led to an exponential increase in the size and complexity of computational models in biology. At the same time, the accelerating pace of progress also highlights limitations in current approaches to modeling. One of these limitations is the insufficient degree to which the semantics and qualitative behaviour of models are systematised and expressed formally enough to support unambiguous interpretation by software systems. As a result, human intervention is required to interpret and connect a model's mathematical structures with information about the its meaning (semantics). Often, this critical information is usually communicated through free-text descriptions or non-standard annotations; however, free-text descriptions cannot easily be interpreted by current modeling tools.
We will describe three efforts to standardise the encoding of missing semantics for kinetic models. The overall approach involves connecting model elements to common, external sources of information that can be extended as existing knowledge is expanded and refined. These external sources are carefully managed public, free, consensus ontologies: the Systems Biology Ontology (SBO), the Kinetic Simulation Algorithm Ontology (KiSAO), and the Terminology for the Description of Dynamics (TeDDy). Together they provide a means for annotating a model with stable and perennial identifiers which reference machine readable regulated terms defining the semantics of the three facets of the modeling process 1) the relationship between the model and the biology it aims to describe, 2) the process used to simulate the model and obtain expected results, and 3) the results themselves.
Yannis Kalaidzidis, Max Planck Institute of Molecular Cell Biology and Genetics, Germany
Quantitative Microscopy: bridge between "wet" biology and computer science
Quantification of experimental evidence is an important aspect of modern life science. In microscopy, this causes a shift from pure presentation of "supporting cases" toward the quantification of the processes under study. Computer image processing breaks through the light microcopy diffraction limit, it allows to track individual molecules in the life specimen, quantify distribution and co-localization of compartment markers, etc. The quantified experimental data forms a basis for the models of the biological processes. Quality of predictive models is crucially dependent on the accuracy of the quantified experimental data. The quality of experimental data is a function of algorithms as well as the imperfections of the "wet" experiment. The number of research papers devoted to the algorithms of microcopy image analysis, segmentation, classification and tracking has grown very fast in the last two decades. The analysis of the source of noise in "wet" biology and microscopy has gotten less attention. In this talk I will focus on the correction of experimental data before applying analysis algorithms. These corrections have two faces. They are obligatory to compensate for imperfections of "wet" microscopy while at the same time this correction can break some assumptions, which form the basis of algorithms for subsequent analysis. The examples of the different approaches for "pre-" and "post-" correction will be presented.
Ross D. King, Aberystwyth University, UK
On the Automation of Science
The basis of science is the hypothetico-deductive method and the recording of experiments in sufficient detail to enable reproducibility. We report the development of the Robot Scientist "Adam" which advances the automation of both. Adam has autonomously generated functional genomics hypotheses about the yeast Saccharomyces cerevisiae, and experimentally tested these hypotheses using laboratory automation. We have confirmed Adam's conclusions through manual experiments. To describe Adam's research we have developed an ontology and logical language. The resulting formalization involves over 10,000 different research units in a nested tree-like structure, ten levelsdeep, that relates the 6.6 million biomass measurements to their logical description. This formalization describes how a machine discovered new scientific knowledge. Describing scientific investigations in this way opens up new opportunities to apply machine learning and data-mining to discover new knowledge.
William Stafford Noble, University of Washington, USA
Machine learning methods for protein analyses
Computational biologists, and biologists more generally, spend a lot of time trying to more fully characterize proteins. In this talk, I will describe several of our recent efforts to use machine learning methods to gain a better understanding of proteins. First, we tackle one of the oldest problems in computational biology, the recognition of distant evolutionary relationships among protein sequences. We show that by exploiting a global protein similarity network, coupled with a latent space embedding, we can detect remote protein homologs more accurately than state-of-the-art methods such as PSI-BLAST and HHPred. Second, we use machine learning methods to improve our ability to identify proteins in complex biological samples on the basis of shotgun proteomics data. I will describe two quite different approaches to this problem, one generative and one discriminative.
The following papers are accepted for oral presentation (ordered by first authors' lastname):
- Antti Airola, Tapio Pahikkala, Willem Waegeman, Bernard De Baets and Tapio Salakoski.
A comparison of AUC estimators in
small-sample studies
- Nicolo' Cesa-Bianchi and Giorgio Valentini.
Hierarchical cost-sensitive algorithms for genome-wide gene function prediction
- Gabor Hullam, Peter Antal, Csaba Szalai and Andras Falus.
Evaluation of methods in GA studies: yet another case for
Bayesian networks
- Zerrin Isik, Volkan Atalay and Rengul Cetin-Atalay.
Evaluation of Signaling Cascades Based on the Weights from
Microarray and ChIP-seq Data
- Wei Liu and Mahesan Niranjan.
Matching models to data in modelling morphogen diffusion
- Minca Mramor, Marko Toplak, Gregor Leban, Tomaz Curk, Janez Demsar and Blaz Zupan.
On utility of gene set signatures
in gene expression-based cancer class prediction
- Malik Sajjad Ahmed Nadeem, Jean-Daniel Zucker and Blaise Hanczar.
Accuracy-Rejection Curves (ARCs) for Comparison
of Classification Methods with Reject Option
- Hossein Rahmani, Hendrik Blockeel and Andreas Bender.
Predicting the functions of proteins in PPI networks from global information
- Matteo Re and Giorgio Valentini.
Simple ensemble methods are competitive with state-of-the-art data integration methods
for gene function prediction
- Yvan Saeys, Sofie Van Landeghem and Yves Van de Peer.
Integrated network construction using event based text mining
- Ivica Slavkov, Bernard Zenko and Saso Dzeroski.
Evaluation Method for Feature Rankings and their Aggregations for
Biomarker Discovery
- Lan Umek, Petra Kaferle, Mojca Mattiazzi, Ales Erjavec, Crtomir Gorup, Tomaz Curk, Uros Petrovic and Blaz Zupan.
A
Subgroup Discovery Approach for Relating Chemical Structure and Phenotype Data in Chemical Genomics
The following posters will be presented (ordered by first authors' lastname):
- Thomas Abeel, Thibault Helleputte, Pierre Dupont and Yvan Saeys. Robust biomarker identification for cancer diagnosis using ensemble feature selection methods
- Thomas Abeel, Yves Van de Peer and Yvan Saeys. Java-ML a Java Library for Data Mining
- Adel Aloraini, James Cussens and Richard Birnie. Extending KEGG Pathways for a Better Understanding of Prostate Cancer Using Graphical Models
- Peter Antal, G. Hajos, A. Millinghoffer, G. Hullam, Cs. Szalai and A. Falus. Variable Pruning in Bayesian Sequential Study Design
- Peter Antal, A. Millinghoffer, Cs. Szalai and A. Falus. On the Bayesian applicability of graphical models in genome-wide association studies
- Peter Antal, P. Sarkozy, B. Zoltan, P. Kiszel, A. Semsei, Cs. Szalai and A. Falus. Averaging over measurement and haplotype uncertainty using probabilistic genotype data
- Matthias Bock, Soichi Ogishima, Lars Kaderali and Stefan Kramer. Bayes Meets Boole: Bayesian Learning of Boolean Regulatory Networks from Expression Data
- Celine Brouard, Julie Dubois, Marie-Anne Debily, Christel Vrain and Florence d'Alche-Buc. Statistical relational learning for supervised gene regulatory network inference
- Eduardo Costa, Celine Vens and Hendrik Blockeel. Top-down phylogenetic tree reconstruction: a decision tree approach
- Bertrand De Meulder, Benoit De Hertogh, Fabrice Berger, Anthoula Gaigneaux, Michael Pierre, Eric Bareke and Eric Depiereux. Using biological data to benchmark microarray analysis methods
- Kristina Gruden, Petra Kralj Novak, Igor Mozetic, Vid Podpecan, Matjaz Hren, Helena Motaln, Marko Petek and Nada Lavrac. Structural modeling of transcriptomics data using creative knowledge discovery
- Giorgio Guzzetta, Giuseppe Jurman and Cesare Furlanello. Phenotype Prediction from Genotype Data
- Thibault Helleputte and Pierre Dupont. Biomarker Selection by Transfer Learning with Linear Regularized Models
- Dimitar Hristovski, Andrej Kastrin, Borut Peterlin and Thomas Rindflesch. Combining Semantic Relations from the Literature and DNA Microarray Data for Novel Hypotheses Generation
- Ilkka Huopaniemi, Tommi Suvitaival, Janne Nikkila, Matej Oresic and Samuel Kaski. Two-Way Analysis of High-Dimensional Metabolomic Datasets
- Agnieszka Lawrynowicz and Ross King. The Open and Closed-World Assumptions in Representing Systems Biology Knowledge
- Fabian Ojeda, Marco Signoretto, Bart De Moor and Johan Suykens. Learning gene networks with sparse inducing estimators
- Michael Pierre, Anthoula Gaigneaux, Bertrand DeMeulder, Fabrice Berger, Benoit DeHertogh, Eric Bareke, Carine Michiels and Eric Depiereux. Taking advantage of the amount of archived Affymetrix GeneChips to identify genes involved in metastasis and regulated by hypoxia
- Bogdan Pogorelc, Jesus Brezmes and Matjaz Gams. Metabolic syndrome assessment using Fuzzy Artmap neural network and 1H NMR spectroscopy
- Barbara Szomolay. Modeling phagocytosis - PHAGOSYS project outline
- Ljupco Todorovski and Saso Dzeroski. Inductive Process-Based Modeling of Endocytosis from Time-Series Data
- Bernard Zenko, Jan Struyf and Saso Dzeroski. Analyzing time series gene expression data with predictive clustering rules
|
|
|
Saturday, 5 September
|
|
08:00 |
17:00 |
|
Registration desk open
|
|
08:50 |
09:00 |
|
Opening
|
|
09:00 |
10:00 |
|
Invited talk
|
|
|
|
|
Diego di Bernardo. Networking Genes and Drugs: Understanding Drug Mode of Action and Gene Function from Large-scale Experimental Data
|
|
10:00 |
10:50 |
|
Session 1: Networks and function
|
|
10:00 |
10:25 |
|
Hossein Rahmani, Hendrik Blockeel and Andreas Bender. Predicting the functions of proteins in PPI networks from global information
|
|
10:25 |
10:50 |
|
Yvan Saeys, Sofie Van Landeghem and Yves Van de Peer. Integrated network construction using event based text mining
|
|
10:50 |
11:20 |
|
Coffee break
|
|
11:20 |
12:20 |
|
Invited talk
|
|
|
|
|
Yannis Kalaidzidis. Quantitative Microscopy: Bridge Between "Wet" Biology and Computer Science
|
|
12:20 |
13:10 |
|
Session 2: Biomarkers and disease
|
|
12:20 |
12:45 |
|
Minca Mramor, Marko Toplak, Gregor Leban, Tomaz Curk, Janez Demsar and Blaz Zupan. On utility of gene set signatures in gene expression-based cancer class prediction
|
|
12:45 |
13:10 |
|
Ivica Slavkov, Bernard Zenko, and Saso Dzeroski. Evaluation Method for Feature Rankings and their Aggregations for Biomarker Discovery
|
|
13:10 |
15:10 |
|
Session 3: Posters and lunch
|
|
13:10 |
15:10 |
|
Poster presentations with catered lunch and coffee.
|
|
15:10 |
16:00 |
|
Session 4: Development, signalling
|
|
15:10 |
15:35 |
|
Wei Liu and Mahesan Niranjan. Matching models to data in modelling morphogen diffusion
|
|
15:35 |
16:00 |
|
Zerrin Isik, Volkan Atalay and Rengul Cetin-Atala. Evaluation of Signaling Cascades Based on the Weights from Microarray and ChIP-seq Data
|
|
16:00 |
17:00 |
|
Invited talk
|
|
|
|
|
Roman Jerala. Synthetic Biology: Achievements and Prospects for the Future
|
|
17:00 |
|
|
Community meeting
|
|
19:00 |
|
|
Guided walk through Ljubljana
|
|
20:00 |
|
|
Conference dinner
|
|
|
|
|
Sunday, 6 September
|
|
09:00 |
10:00 |
|
Invited talk
|
|
|
|
|
William Stafford Noble. Machine Learning Methods for Protein Analyses
|
|
10:00 |
10:50 |
|
Session 5: Machine learning methodology
|
|
10:00 |
10:25 |
|
Antti Airola, Tapio Pahikkala, Willem Waegeman, Bernard De Baets and Tapio Salakoski. A comparison of AUC estimators in small-sample studies
|
|
10:25 |
10:50 |
|
Malik Sajjad Ahmed Nadeem, Jean-Daniel Zucker and Blaise Hanczar. Accuracy-Rejection Curves (ARCs) for Comparison of Classification Methods with Reject Option
|
|
10:50 |
11:20 |
|
Coffee break
|
|
11:20 |
12:20 |
|
Invited talk
|
|
|
|
|
Nick Juty. Ontologies for Systems Biology
|
|
12:20 |
13:10 |
|
Session 6: Function prediction
|
|
12:20 |
12:45 |
|
Nicolo' Cesa-Bianchi and Giorgio Valentini. Hierarchical cost-sensitive algorithms for genome-wide gene function prediction
|
|
12:45 |
13:10 |
|
Matteo Re and Giorgio Valentini. Simple ensemble methods are competitive with state-of-the-art data integration methods for gene function prediction
|
|
13:10 |
15:10 |
|
Session 7: Posters and lunch
|
|
13:10 |
15:10 |
|
Poster presentations with catered lunch and coffee.
|
|
15:10 |
16:00 |
|
Session 8: Phenotype prediction
|
|
15:10 |
15:35 |
|
Lan Umek, Petra Kaferle, Mojca Mattiazzi, Ales Erjavec, Crtomir Gorup, Tomaz Curk, Uros Petrovic and Blaz Zupan. A Subgroup Discovery Approach for Relating Chemical Structure and Phenotype Data in Chemical Genomics
|
|
15:35 |
16:00 |
|
Gabor Hullam, Peter Antal, Csaba Szalai and Andras Falus. Evaluation of methods in GA studies: yet another case for Bayesian networks
|
|
16:00 |
17:00 |
|
Invited talk
|
|
|
|
|
Ross D. King. On the Automation of Science
|
|
17:00 |
|
|
Discussion and Closing remarks
|
|
18:00 |
|
|
Shuttle to Bled (for ECML/PKDD participants)
|
|
|
|
|